Autonomous driving is no longer a concept confined to science fiction. In recent years, significant strides have been made to bring self-driving technology to our streets. Companies like Tesla, Waymo, and Apple are pouring billions into the development of vehicles that can drive with minimal or no human intervention. The driving force behind this revolution isn’t just advanced mechanical engineering but a robust synergy of sensor technologies and software algorithms.
Understanding autonomous vehicles means diving deep into how they interpret their surroundings, make decisions, and execute actions safely and efficiently. This blog post explores the crucial elements that power self-driving cars: sensor technologies and software algorithms, examining their roles, challenges, and future implications.
The Levels of Autonomy in Vehicles
Autonomous driving is classified into six levels by the Society of Automotive Engineers (SAE), ranging from Level 0 (no automation) to Level 5 (full automation). Each level represents an increase in a vehicle’s capability to perform driving tasks without human intervention.
Level 0 and 1 vehicles still require full human control or offer minimal assistance, such as cruise control. Levels 2 and 3 introduce partial automation with features like adaptive cruise control and lane-keeping assistance. At these stages, drivers must remain alert and ready to take over at any time. Level 4 allows vehicles to operate without human input in specific conditions, while Level 5 represents complete autonomy in all driving environments.
This classification helps set clear expectations for both developers and consumers. It also establishes regulatory guidelines that influence how vehicles are tested and deployed. Understanding these levels provides a foundational framework to appreciate the complexity involved in autonomous driving.
Key Sensors Used in Autonomous Vehicles
Sensor technologies form the eyes and ears of an autonomous vehicle. These sensors collect real-time data about the car’s environment, enabling the onboard computer to make informed decisions. The primary sensors include LiDAR, radar, cameras, ultrasonic sensors, and GPS.
LiDAR (Light Detection and Ranging) uses laser beams to create a 3D map of the surroundings. It is particularly effective in detecting obstacles and determining their exact position and size. Radar systems are useful for detecting the speed and distance of moving objects, even in poor weather conditions. Cameras offer visual information necessary for interpreting traffic signals, lane markings, and pedestrian activity.
Ultrasonic sensors, often found in bumpers, help with short-range detection, making them ideal for parking assistance. GPS combined with high-definition mapping provides positional accuracy crucial for route planning and navigation. These sensors work together to provide a comprehensive view of the vehicle’s environment, forming the basis for subsequent decision-making processes.
Role of Software Algorithms in Vehicle Autonomy
While sensors gather data, software algorithms are responsible for interpreting that data and converting it into actionable decisions. These algorithms are the brain of autonomous vehicles, processing vast amounts of information in real-time to navigate safely and efficiently.
Software algorithms handle a range of tasks, from object detection and classification to motion planning and decision-making. Machine learning models enable the vehicle to identify pedestrians, cyclists, and other vehicles. Predictive algorithms assess the likely actions of nearby objects, such as a pedestrian preparing to cross the street. This predictive capacity is vital for avoiding accidents and ensuring smooth traffic flow.
Additionally, software algorithms coordinate with control systems to manage steering, acceleration, and braking. Advanced algorithms also perform path planning, which involves choosing the best route based on current traffic, road conditions, and dynamic obstacles. The continuous refinement of these algorithms, often through real-world data and simulation environments, ensures the vehicle adapts and improves over time.
Sensor Fusion: Integrating Multiple Inputs
Sensor fusion is the process of integrating data from various sensors to create a coherent and accurate understanding of the driving environment. Each sensor type has its strengths and weaknesses; for example, cameras struggle in low light, while radar can’t provide high-resolution images.
By combining data from LiDAR, radar, cameras, and other sensors, sensor fusion algorithms can compensate for individual limitations and enhance overall perception. For instance, while a camera may capture the color of a traffic light, LiDAR can determine its exact position, and radar can detect vehicles approaching the intersection.
Effective sensor fusion improves the reliability of object detection and tracking, especially in complex urban settings where multiple elements interact simultaneously. This holistic approach allows autonomous systems to make safer and more accurate decisions.
Machine Learning and Neural Networks in Autonomous Driving
Machine learning and deep neural networks play a pivotal role in the evolution of autonomous driving. These AI-based technologies allow the system to learn from vast datasets and improve over time without explicit programming for every scenario.
Neural networks are particularly effective in image recognition tasks, such as identifying stop signs, traffic lights, and pedestrians. Convolutional Neural Networks (CNNs) are used to process visual data, enabling the vehicle to understand its environment similar to how a human would.
Reinforcement learning is another technique employed, where algorithms learn optimal behavior through trial and error. Simulation environments provide a safe space for these algorithms to test and refine their strategies. The continuous development of machine learning models ensures that autonomous systems become more robust and adaptable to varying driving conditions.
Real-Time Decision Making and Planning
Autonomous vehicles must make decisions in real-time, often under complex and unpredictable conditions. This includes responding to sudden obstacles, interpreting hand signals from pedestrians, or rerouting due to road closures.
Software algorithms designed for real-time decision making rely on rapid data processing and efficient resource management. These algorithms prioritize tasks, manage system resources, and ensure time-sensitive actions are executed promptly. The ability to process sensor inputs, evaluate scenarios, and determine the best course of action within milliseconds is what sets high-performing autonomous systems apart.
Motion planning algorithms also contribute to real-time performance. They calculate the most efficient and safe trajectory for the vehicle, taking into account current speed, road curvature, and nearby objects. The integration of these planning algorithms with control systems ensures that the vehicle can execute complex maneuvers smoothly and safely.
Challenges in Sensor and Software Integration
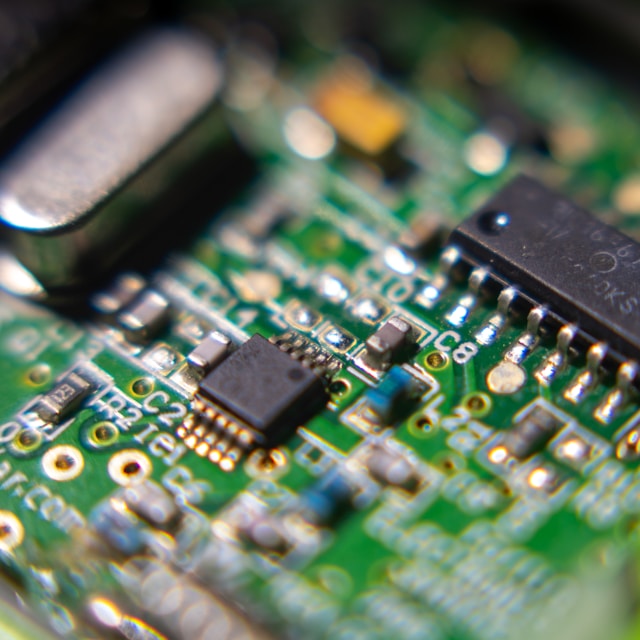
Despite remarkable progress, integrating sensors and software algorithms presents several challenges. One major issue is ensuring accuracy and reliability across different sensor modalities. Environmental factors like rain, fog, or snow can impair sensor performance, leading to potential misinterpretations.
Software algorithms must also be resilient to unexpected scenarios. Edge cases, such as unusual pedestrian behavior or obscure road markings, require systems to make decisions without clear precedents. This necessitates highly generalized learning models and extensive testing.
Moreover, real-time processing demands significant computational power, which can strain onboard systems. Balancing performance with energy efficiency is a persistent challenge. Redundancy and fail-safe mechanisms must also be in place to handle system failures gracefully, ensuring passenger safety at all times.
Testing and Validation of Autonomous Systems
Before autonomous vehicles can be widely deployed, they must undergo rigorous testing and validation. This process involves both virtual simulations and real-world driving tests to ensure system reliability under diverse conditions.
Simulations allow developers to test software algorithms in millions of scenarios quickly and cost-effectively. They also enable training for rare but critical edge cases. However, real-world testing remains indispensable for evaluating how the vehicle interacts with dynamic environments and human behavior.
Validation also includes compliance with regulatory standards. Governments and industry bodies require detailed documentation, performance metrics, and safety assurances before approving autonomous systems for public roads. The combination of simulation, road testing, and regulatory review helps ensure that self-driving cars are safe and trustworthy.
The Future of Autonomous Vehicle Technologies
Looking ahead, the future of autonomous driving is bright but still under development. Innovations in sensor miniaturization, more efficient software algorithms, and increased computational capabilities will further enhance vehicle performance.
Emerging technologies like quantum computing and 5G connectivity may also play a role. Quantum processors could drastically reduce processing time for complex calculations, while 5G enables low-latency communication between vehicles and infrastructure. These advancements could facilitate more cooperative and intelligent transportation systems.
Autonomous vehicles will likely evolve from individual machines into interconnected nodes in a smart mobility ecosystem. These systems will communicate with traffic signals, other vehicles, and cloud services to optimize traffic flow, reduce emissions, and improve safety. The integration of software algorithms and sensor technologies will continue to be the cornerstone of this transformation.
Conclusion: The Road Ahead
Autonomous driving represents a paradigm shift in transportation, promising greater safety, efficiency, and accessibility. The seamless integration of sensor technologies and software algorithms is what makes self-driving vehicles possible. Each component, from LiDAR to machine learning models, plays a vital role in ensuring that these vehicles can navigate the world autonomously.
While challenges remain, the continued advancement in both hardware and software signals a future where autonomous vehicles are a common sight on our roads. As research and development continue, the role of software algorithms in managing and interpreting data will become even more critical. In this rapidly evolving landscape, understanding the underlying technology is key to appreciating how we are redefining the way we move.
Stay tuned for more insights into the fascinating world of autonomous driving and the technologies powering the journey to full automation.